On artificial neurological illnesses
As AIs gain reach and complexity, unexpected & unkown behaviours shall appear. What do we do with that?
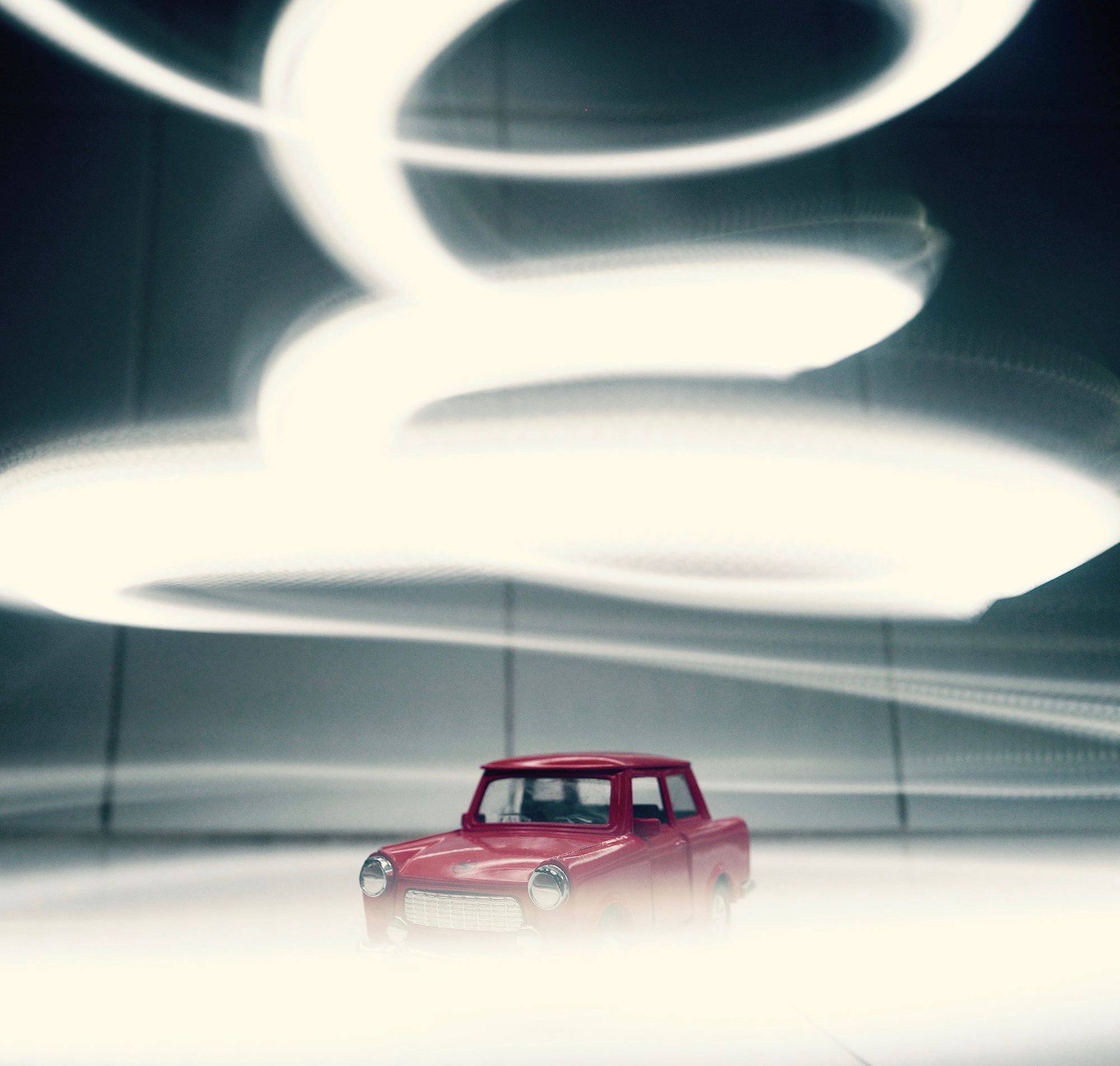
(originally posted on Medium in 2018, republished in my own site two years after that)
Frontiers are host to the most interesting combinations of life.
Where are the frontiers for normal human behaviour? What is personality, what is illness? What makes us go beyond those frontiers?
In his book “the man who mistook his wife for a hat”, a compilation of several neurological case histories recounted with exquisite style, wit and compassion, Oliver Sacks told the story of a Dr. P who suffered from visual agnosia (the inability to make sense of what you see). Dr. P was a music professor and renowned musician who understood the world around him through music and visual input. He resorted to a sort of rhythm in his activities (getting dressed, eating lunch) or in his context (the way someone walks) to recognize those activities and to know what he had to do. As Dr. Sacks writes in his book: “He sings all the time — eating songs, dressing songs, bathing songs, everything. He can’t do anything unless he makes it a song”. Dr. P could not recognize a flower, instead producing a rich, detailed description of what he had in front of him (“About six inches in length, a convoluted red form with a linear green attachment.”). Until he smelled it (“Beautiful! An early rose. What a heavenly smell!”). He could not make sense of what was on his left field of view, but had no trouble in living life in this way: he was not aware that there was anything wrong in the way he perceived the world around him. Although he would halt to a complete stop when his “inner music” didn’t flow, he wouldn’t think of that as something out of the ordinary, as something that needed attention. That is, he was quite unconscious of what was going on.
Human cognition is still largely a mystery, but it seems from what we know that our capacity to understand our context and adapt to it has to do with the tight and intimate integration of several complex systems. In the case of vision, following from the previous story, millions of stimuli travel from the retina to the cortical areas related to vision; they are processed in several layers at different depths in the visual cortex, and the output of each layer is then connected to many other areas of the brain which are related to several functions: areas dealing with semantics, memory, emotions, planning, motricity, etc. The same holds true for auditory stimuli, olfactory and so on. Memories too. The brain interconnects and processes all these stimuli in real-time. Emotions, behaviour and a sense of being emerge out of all that.
But what happens if a number of connections fail? When a bunch of neurons act different than normal? When a neurotransmitter can’t be produced? Or when one of your senses works suddenly different, that is, when the stimuli are unexpected? Oliver Sacks presents brilliantly in his entire writing production many such cases. Perceptions of reality where something is missing. Perceptions that are hard to describe in words and hard to imagine from a first-person standpoint for someone without those conditions. Neurological illnesses emerge, much as consciousness itself, from the interplay of several systems performing relatively simple tasks and where one or more elements are slightly off. And it’s not just altered perceptions of reality. It’s a very wide spectrum of modes of functioning, some of which we identify as illness, some we identify as “types of personality”, some as mere anecdotes in a person’s life. Distinguishing among illness, odd behaviour and strong personalities is beyond the scope of this article and deserves a paused reflection.
Just as there is a wide array of perceptions of reality and behaviours in us living entities, products and services today start to exhibit their own wide array of behaviours, thanks to artificial intelligence (AI) systems that we humans bake into them. For the sake of simplicity, let me use AI as a broad term going from simple machine learning techniques to complex collections of algorithms operating in unison.
Almost every respectable digital product and industry has some sort of it. What to listen to next? Spotify, Pandora will make a pretty nice guess at something you might like and that’s, well, a simple form of AI (a machine learning recommendation system, in this case). Where to eat today? There’s a bunch of apps squeezing their algorithms for a suggestion that you’ll like. How to coordinate the ground staff at an airport to serve as many planes as possible in today’s modern, busy airports? There’s an AI for that, and for medical services, energy distribution systems and almost everything you can think about. And it’s great, since AI enables new products, greater efficiencies, scaling services to the world population and so on. That is, when they are healthy.
We are at the early stages of a long history of the evolution of AI systems. Their behaviours are simplistic, their contextual interaction scopes are limited. The consequences of ill or bad behaviour are also limited. But as systems get more complex, so do their interaction scopes and their behaviours, and in turn the potential implications of these becoming strange or unexpected. How do these future systems look like? As nervous systems increase in complexity, we go from simple amoeba all the way to us humans. As AI systems increase in complexity, we get… what do we get?
Self-driving cars. Self-flying and self-operating cameras that intelligently follow you and pick the point of view to create unique memories of your life. Machines that can phone a restaurant to book a table. Systems that aid doctors in real time highlighting the tumours with AR during surgery and providing feedback. These are what we already have. Where will we go from here? Hybrid human-AI systems that help us “see” the connections of what lies in front of us. Machines that run companies autonomously. Air travel 100% controlled by AIs. Intelligent teapots. A sofa that warms you up when you are alone feeling a bit blue. Whatever you can imagine.
Much like neurological illnesses emerged as a consequence of the increasing complexity in our nervous systems, artificial neurological illnesses will emerge in the increasingly complex AI systems we build. And they will for the same reason: unexpected connections with unexpected consequences in systems of growing complexity.
Artificial neurological illnesses are not a classical bug per se. They may be triggered by a classical bug, some may be triggered by unexpected sensory inputs. Some by the history of live data / context they need to adapt to. Some by unexpected interactions among the different systems that make up the AI in question. These are what fascinate me. We will witness unexpected behaviours, emerging behaviours which we didn’t code and we didn’t train those systems for. Behaviours which seem unreasonable for the task that those AIs were created for. Odd behaviours. Perhaps something like these:
Artificial autism? Autistic brains display tightly interconnected local areas, and poor connection patterns between areas that are apart in the brain. How could it happen? An AI composed of several models working together, where the learning rates are not properly tuned, may end up exhibiting a similar pattern. The model that connects the different models together may assign few dependencies among them, low values to their coupling variables. Some of the individual models may start to overfit. An autistic self-driven car may end up paying attention only to traffic lights and nothing else, and drive in some certain area and refuse to go anywhere else. Was Uber’s self-driving car accident an early sign for this? Failure to consider all the inputs it had, focusing instead on just part of the information available? (we know that was not the case, but it could be soon).
Artificial paranoia? Some dirt in the camera that feeds images to a vision and reasoning engine may trigger unexpected results from the vision system that propel the reasoning system to a wild understanding of what’s around it. And if this system is connected to some other system that is doing some kind of unsupervised learning, the effects become long-lasting in the system. The self-flying camera starts seeing obstacles where they are not and chooses to fly into a tree.
Artificial hallucinations? A weather prediction algorithm managing a renewable energy plant that encounters a period of strangely low wind activity and its feedback loops are not trained for that. It starts hallucinating predictions that make no sense, causing an incorrect balance in the energy network. We’ve seen the flash crash in the financial markets, was that an early symptom?
Artificial depression? A Google Duplex that picks up reservation calls in a restaurant asking people why do they want to go out for dinner when life sucks.
The current evolution of AI does not point at a near future full of flourishing general artificial intelligence. We probably will not see natural language algorithms embedded in Alexa or Google Now or any other such service developing Tourette syndrome and exhibiting vocal tics or even coprolalia (becoming mouth-fouled, “there’s heavy traffic in your area, you’ll suffer a 10 minute delay, f**k you”). In the short term, however, we may find a growing number of cases where we can’t really explain why your autonomous car has a tendency to scratch other cars bumpers when parking, when it never did so when you started your mutual relationship. We’ve already witnessed how chatbots may drift completely outside of what they were meant to be doing: Microsoft´s Tay infamous incident is the beginning of a journey into teenage AIs which have a personality disorder. It may develop Tourette’s at some point.
How these artificial neurological illnesses play out in the real world is difficult to predict. The wide range of AI skills and capability level makes it basically a one-by-one review case. When our machines develop these ailments, can we teach our AIs to stop and wonder what is going on? To stop execution if they don’t know what they are doing? If they develop the same condition as Dr. P in Oliver Sack’s story, perhaps they can’t.
This calls for a new perspective on these matters. Humans developed the sciences of psychology, psychiatry, neurology, etc. Maybe we need to train a branch of our current crop of data scientists in the science of machine psychology, machine neurology and machine psychiatry. Artificial neurologists, artificial psychologists, artificial psychiatrists. With Machine Learning we have now… machines that are learning. Maybe soon it will be the time to learn about those learning machines. Because at some point they do evolve somehow out of our control.
What can we do today? Some ideas:
1) Improve education, raise awareness. There’s already plenty of (necessary) talk about caring about the biases that we inject into our algorithms, the ethical dimension of how we apply these algorithms, which problems we apply them to, which are the changes in behaviour that we instill in society because of how the algorithm is trained, etc. This list could add this concept of caring for the mental sanity of our AIs. Mental health checks. Perhaps something like the “empathy test” that was applied to replicants in Blade Runner, but designed not to test for “replicant-ness” but rather to test for proper algorithm health (there are some notable proposals that check for bias, for example the Turing Box; also there are organizations such as Algorithm Watch, but these are all focused on ethics / bias / etc., not yet on proper algorithmic health).
2) Establish best practices where algorithms are published / released always hand in hand with the set of assumptions made, the training set of data used, the test set of data used, the learning metrics, the cost functions, etc. so an artificial neuropsychiatrist or artificial neurologist can have a better understanding of the potential underlying pathology. And how the different systems are interconnected (since we are talking about products / services with several interwoven AIs working together). Which scenarios they are prepared to deal with. By the way, it would be great to have these for humans! So we would know what to expect from ourselves.
2) Design systems that minimize these effects, perhaps by rejecting non-interpretable approaches. But they are so appealing with their current performance… Or design systems in a way that is easy to perform some sort of artificial magnetic functional resonance, for example: some procedure that lets us “see” into how the algorithm is working right now.
3) Employ a holistic approach to your digital product design: use people who know people and machines to understand where are the new relationships, the implications, everything. This can prepare us to design better for potential algorithmic mental health problems.
4) Avoid digital! Ask your friends what to listen to and where to eat, drive your own car. Love the people you love, instead of spending too much time with your machines.
This will all happen some time from now. Or not. On the meantime, going back to the opening question, where are the frontiers for normal behaviour? It ends where love begins. But how that applies to AIs is a topic for a different article.